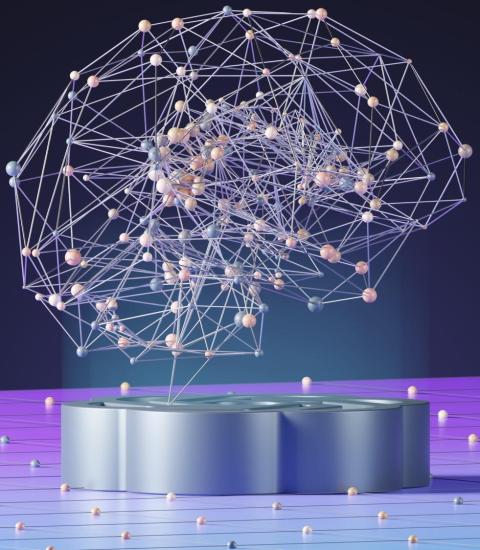
Call for Papers for a Special Issue of Information Polity
Generative AI in Public Service
Special Issue Guest Editors
- Adegboyega Ojo, School of Public Policy and Administration, Faculty of Public Affairs, Carleton University, Ottawa, Canada, adegboyega.ojo@carleton.ca
- Sehl Mellouli, Department of Management Information Systems, Faculty of Business Administration, Laval University, Quebec, Canada
- Kasia Polanska, School of Public Policy and Administration, Faculty of Public Affairs, Carleton University, Ottawa, Canada
- Nina Rizun, Department of Informatics in Management, Gdansk University of Technology, Poland
Artificial intelligence (AI) garnered significant public attention following the 2022 launch of ChatGPT. This Generative AI chatbot, built on a Large Language Model (LLM), is engineered to process natural language efficiently handle vast text data, discern intricate patterns within text, and yield insightful outputs expressed in natural languages (Council of the European Union, 2023). LLM-based applications in the public sector can support a variety of administrative and decision making tasks (Cantens, 2024; Gao, 2023). These include translation of administrative documents, summarisation of documents for briefing, 24/7 conversational assistants to support citizen enquiries, and analysis of policy or administrative documents. With their potential for significant efficiency gains, LLMs are being increasingly integrated into public sector applications. Nonetheless, this integration is not without its challenges, raising critical questions and concerns that governments and public services must address.
The introduction of LLMs in the public sector challenges all tenets of government, from its legal foundation through the character of the work of a public servant to the provision of the most straightforward services. No other technology has had such a transformative impact on the government. It comes with benefits and tremendous opportunities, but its introduction also necessitates a careful balance of public values - such as confidentiality, transparency, fairness, and accountability - with experimentation and innovation (Berryhill et al., 2019; Cantens, 2024; Council of the European Union, 2023; Giest & Grimmelikhuijsen, 2020; Straub et al., 2023). Governments face complex decisions in implementing LLMs with responsibility stemming from their multifaceted function as LLMs’ financiers, regulators, and users, each role demanding a nuanced understanding of the technology and its implications (Berryhill et al., 2019; Ubaldi et al., 2019), requiring an analysis of alternatives and trade-offs based on the current understanding of technology, which might be lacking given its complexity and limited access to expertise.
As LLMs become introduced and applied to various settings within public service and beyond, governments race to establish rules that provide support and direction while often aiming to create enough space for flexibility and experimentation. Through regulations, governments might also ensure that it is used to promote and protect societal goals and values (Berryhill et al., 2019; Cantens, 2024; Christian, 2020; Straub et al., 2023). This might include fostering diverse and inclusive perspectives to mitigate social and environmental injustices (Okerlund et al., 2022).
While LLMs offer substantial benefits, they also pose significant challenges. Trained on historical data, they can perpetuate biases related to race, gender, and religion (Gao, 2023; Gover, 2023; Okerlund et al., 2022). Additionally, their susceptibility to errors and misinformation is a critical concern, with larger systems potentially amplifying biases (Zhang et al., 2022). To introduce these applications in public service, they must be accompanied by standard operational procedures and clear epistemic criteria (Straub et al., 2023). Governments must also establish rules for using LLMs in other sectors, balancing innovation, public trust and private sector involvement (Giest & Grimmelikhuijsen, 2020). Corporate efforts to commercialize LLMs have sparked questions about copyright and privacy, monopolization of technology, and dependence on large-scale computing power (Luitse & Denkena, 2021). Academic researchers often rely on the private sector for LLM access, creating partnerships with companies in this field and exacerbating potential power imbalances between the private sector, governments and society, highlighting the need for careful oversight (Okerlund et al., 2022; Stokel-Walker & Van Noorden, 2023).
LLMs can also alter labour structures by reconfiguring tasks and job descriptions, automating certain tasks, and freeing civil servants’ time for more complex and high-priority activities (Council of the European Union, 2023). Effective implementation among public servants requires critical thinking and adapting to changing demographics (Agrawal et al., 2023; Berryhill et al., 2019; Cantens, 2024; Korzynski et al., 2023). The global research landscape reflects a burgeoning interest in the application of LLMs in public service. Studies bring other areas of inquiry, including examinations of the usability of LLM in a public sector context (e.g. Peña et al., 2023), investigations of the adoption of AI in public sector organizations (Medaglia et al., 2023; Selten & Klievink, 2024; van Noordt & Misuraca, 2022), particular impacts of LLMs in developing countries, and related hegemonic tendencies (Mannuru et al., 2023), as well as a growing body of research on the social impact, ethics, and regulation of machine learning systems (Luitse & Denkena, 2021). Generative AI has also been seen as a new context, leading to the emergence of new management theories and concepts (Korzynski et al., 2023).
Developing a conceptual and theoretical understanding of LLMs in Public Service is essential. Doing so will allow for a new area of scholarly inquiry that responds to calls to formalize the study of AI in the public sector (Straub et al., 2023).
Scope of the Special Issue
This special issue will be transdisciplinary, acknowledging the breadth of knowledge and insight needed to untangle the overarching theme of the special issue. Submitted papers are expected to draw on expertise from public administration, information systems, public management, science, technology, society studies, and philosophy, among others. We welcome submissions from any discipline, theoretical, or philosophical perspective and especially encourage authors and papers from underrepresented communities and geographic locations. Manuscripts can be theoretical or empirical but must be methodologically rigorous and contribute to our understanding of using LLMs in the public sector. Published manuscripts are expected to be approximately 8,000 words in length and address questions like the following:
- How are LLMs used in public service, and what are the expected outcomes, efficiencies, and consequences?
- What effects and impacts, if any, do LLMs have on the public service?
- How do LLMs influence decision-making processes in government, and what are the implications for governance and accountability?
- How and under which conditions can LLMs be successfully integrated into public service operations
- What role do regulatory and policy frameworks play in the development and use of large language models?
- What are the primary considerations and potential applications for large language models (LLMs) that policymakers and public sector leaders should focus on to ensure ethical usage, maximized public benefit, and minimized risk to citizens?
- How do citizens feel about the introduction of LLMs in public services? How can LLMs be utilized in public service to promote social and environmental justice, and what role do they play in mitigating injustices?
- How do LLMs transform labour structures within public service, and how does this affect management and governance?
- Considering LLMs’ susceptibility to errors and misinformation, what strategies can be implemented in the public sector to mitigate these risks?
- How can governments balance the need for innovation in AI with maintaining public trust and ethical standards in the public service?
- What empirical evidence exists regarding the usability and effectiveness of LLMs in public sector contexts?
Important dates for the publication of this special issue are as follows:
Deadline abstract submission: October 1st 2024
Invitation to submit full paper: November 1st 2024
Deadline submission full manuscript: February 1, 2025
Review process: February 1st – July 1st, 2025
Final decision on manuscripts: September 2025
Anticipated publication: Issue 1, 2026
Abstracts should initially be sent to Adegboyega Ojo adegboyega.ojo@carleton.ca. Abstracts should be up to 750 words and include the names of all authors and their institutional affiliations.
Abstracts will be reviewed by the Guest Editors of the Special Issue. This review will focus on the fit with the special issue theme, feasibility, and potential contribution to knowledge. The authors of accepted abstracts will be invited to submit full manuscripts. Full manuscripts will be double-blind peer reviewed. Please note that initial acceptance of an abstract does not guarantee acceptance and publication of the final manuscript.
Final manuscripts have to be submitted directly through Information Polity’s submission system and needs to adhere to the journals submission guidelines: www.informationpolity.com/guidelines
About Information Polity
Information Polity is a tangible expression of the increasing awareness that Information and Communication technologies (ICTs) have become of deep significance for all polities as new technology-enabled forms of government, governing and democratic practice are sought or experienced throughout the world. This journal positions itself in these contexts, seeking to be at the forefront of thought leadership and debate about emerging issues, impact, and implications of government and democracy in the information age.
More information: http://informationpolity.com
Author Instructions
Instructions for authors for manuscript format and citation requirements can be found at:
https://informationpolity.com/guidelines
Information Polity Editors-in-Chief
Professor Albert Meijer, Utrecht University
Professor William Webster, University of Stirling
Bibliography
Berryhill, J., Kok Heang, K., Clogher, R., & Mcbride, K. (2019). Hello, World: Artificial intelligence and its use in the public sector. https://doi.org/10.1787/726fd39d-en
Cantens, T. (2024). How will the state think with ChatGPT? The challenges of generative artificial intelligence for public administrations. AI & SOCIETY. https://doi.org/10.1007/s00146-023-01840-9
Christian, B. (2020). The alignment problem: Machine learning and human values (First edition). W.W. Norton & Company.
Council of the European Union. (2023). ChatGPT in the Public Sector-overhyped or overlooked? 1–23.
Giest, S., & Grimmelikhuijsen, S. (2020). Introduction to special issue algorithmic transparency in government: Towards a multi-level perspective. Information Polity, 25(4), 409–417. https://doi.org/10.3233/IP-200010
Korzynski, P., Mazurek, G., Altmann, A., Ejdys, J., Kazlauskaite, R., Paliszkiewicz, J., Wach, K., & Ziemba, E. (2023). Generative artificial intelligence as a new context for management theories: Analysis of ChatGPT. Central European Management Journal, 31(1), 3–13. https://doi.org/10.1108/CEMJ-02-2023-0091
Luitse, D., & Denkena, W. (2021). The great transformer: Examining the role of large language models in the political economy of AI. Big Data and Society, 8(2). https://doi.org/10.1177/20539517211047734
Mannuru, N. R., Shahriar, S., Teel, Z. A., Wang, T., Lund, B. D., Tijani, S., Pohboon, C. O., Agbaji, D., Alhassan, J., Galley, J. Kl., Kousari, R., Ogbadu-Oladapo, L., Saurav, S. K., Srivastava, A., Tummuru, S. P., Uppala, S., & Vaidya, P. (2023). Artificial intelligence in developing countries: The impact of generative artificial intelligence (AI) technologies for development. Information Development. https://doi.org/10.1177/02666669231200628
Medaglia, R., Gil-Garcia, J. R., & Pardo, T. A. (2023). Artificial Intelligence in Government: Taking Stock and Moving Forward. Social Science Computer Review, 41(1), 123–140. https://doi.org/10.1177/08944393211034087
Okerlund, J., Klasky, E., Middha, A., Kim, S., Rosenfeld, H., Kleinman, M., & Parthasarathy, S. (2022). What’s in the Chatterbox? Large Language Models, Why They Matter, and What We Should Do About Them. University of Michigan. https://stpp.fordschool.umich.edu/research/research-report/whats-in-the-chatterbox
Peña, A., Morales, A., Fierrez, J., Serna, I., Ortega-Garcia, J., Puente, Í., Córdova, J., & Córdova, G. (2023). Leveraging large language models for topic classification in the domain of public affairs. Lecture Notes in Computer Science (Including Subseries Lecture Notes in Artificial Intelligence and Lecture Notes in Bioinformatics), 14193 LNCS(Llm), 20–33. https://doi.org/10.1007/978-3-031-41498-5_2
Selten, F., & Klievink, B. (2024). Organizing public sector AI adoption: Navigating between separation and integration. Government Information Quarterly, 41(1), 101885. https://doi.org/10.1016/j.giq.2023.101885
Stokel-Walker, C., & Van Noorden, R. (2023). What ChatGPT and generative AI mean for science. Nature, 614(7947), 214–216. https://doi.org/10.1038/d41586-023-00340-6
Straub, V. J., Morgan, D., Bright, J., & Margetts, H. (2023). Artificial intelligence in government: Concepts, standards, and a unified framework. Government Information Quarterly, 40(4), 101881. https://doi.org/10.1016/j.giq.2023.101881
Ubaldi, B., Le Fevre, E. M., Petrucci, E., & Micheli, P. (2019). State of the art in the use of emerging technologies in the public sector (OECD Working Papers on Public Governance 31; OECD Working Papers on Public Governance, Vol. 31). https://doi.org/10.1787/932780bc-en
van Noordt, C., & Misuraca, G. (2022). Artificial intelligence for the public sector: Results of landscaping the use of AI in government across the European Union. Government Information Quarterly, 39(3), 101714. https://doi.org/10.1016/j.giq.2022.101714