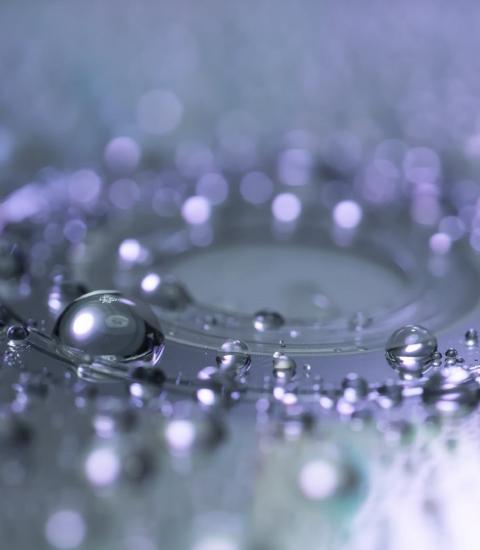
Special Issue: Public Governance and Policy in the Sharing Era
Guest Editors of the Special Issue:
Sarah Giest (Leiden University) & Stephan Grimmelikhuijsen (Utrecht University)
Introduction
Machine-learning technologies and the algorithms that power them hold a huge potential to make government services fairer and more effective, ‘freeing’ decision-making from human subjectivity (Margetts & Dorobantu 2019; Pencheva et al. 2018). Algorithms today are used everywhere from health care to criminal justice; for instance, they can predict recidivism better than criminal court judges (Kleinberg et al. 2017). Research indicates that the introduction of algorithms in decision-making procedures can cause profound shifts in the way bureaucrats make decisions (Peeters and Schuilenberg 2018) and that many government organizations are now starting to use algorithms in decision-making (Statistics Netherlands, 2018).
A major issue with the new generation of algorithms that has been identified by various scholars is the lack of algorithmic transparency (Janssen and Van den Hoven 2015; Kroll et al. 2016). First, the algorithms used in government decisions are often inaccessible because they are developed by commercial parties that consider them intellectual property (Mittelstadt et al. 2016). Second, machine-learning algorithms produce decisional outcomes that may not make sense to people (Burrell 2016). This has been picked up by the conceptual idea of ‘throughput legitimacy’, which, according to Schmidt (2013: 5), is the ‘black box’ of government, the ‘space’ between the political input and the policy output and refers to the engagement of various actors in decision-making processes (i.e. stakeholder and citizen participation). This so-called ‘black box problem’ of algorithmic decision-making makes perceived bias or unfair algorithms hard to scrutinize and contest. This is problematic because when public servants make decisions based on these non-transparent algorithms, this comes at the very real risk of making more biased decisions and eroding public trust in government (Whitakker et al. 2018).
These issues speak to a larger accountability problem when it comes to the use of algorithmic decision-making in that transparency is only achieved in the context of a literate audience. In other words, government departments sharing available documentation, procedures and code does not make decisions more transparent if these elements are not understood by the intended audiences (e.g. citizens, public servants). Some even claim that providing insights can create credibility without proper scrutinizing of the contents (Kemper and Kolkman 2018). In short, signifiers of transparency can incite people’s trust in a system and result in a less critical attitude, but not necessarily in a better procedural set-up.
Given the increasing influence of algorithms in government and the prominence of algorithmic transparency to solve some of the problems associated with the use of algorithms we invite researchers to submit abstracts that investigate this issue. Contribution can be both of conceptual and empirical nature.
- Conceptual clarifications of algorithmic transparency.
- Systematic reviews of existing studies on algorithmic transparency.
- Empirical investigations (quantitative or qualitative) on the effects of algorithmic transparency on citizens and governments
- Barriers of increased algorithmic transparency and how to overcome them.
- Critical perspectives on algorithmic transparency.
- Use of algorithms in public service delivery and transparency linked to discretionary issues
Researchers are invited to submit an abstract of no more than 1,000 words (excluding references). The abstract should outline the theoretical contribution, linked to one of the themes mentioned above, and whether data (if applicable) has been collected or provide an indication of when it will be collected.
Abstracts should be emailed to Sarah Giest s.n.giest@fgga.leidenuniv.nl
Timeline
February 1, 2020: Deadline for abstract submission
February 15, 2020: Notification of decision to submit full manuscript
May 15, 2020: Full manuscript deadline
May-September 2020: Review process (first and possibly second round)
October 1, 2020: Final decision on manuscript
Abstracts will be reviewed by the guest editors of this issue. This review will focus on the fit with the special issue theme, feasibility and potential contribution.
Accepted abstracts will undergo double-blind peer review. Please note that initial acceptance of an abstract does not guarantee acceptance of the full manuscript in any way.
Final manuscripts have to be submitted directly through IP’s submission system and needs to adhere to the journals submission guidelines (https://informationpolity.com/guidelines).
About Information Polity
Information Polity is a tangible expression of the increasing awareness that Information and Communication technologies (ICTs) have become of deep significance for all polities as new technology-enabled forms of government, governing and democratic practice are sought or experienced throughout the world. This journal positions itself in these contexts, seeking to be at the forefront of thought leadership and debate about emerging issues, impact, and implications of government and democracy in the information age.
More information: http://informationpolity.com
References
Burrell, J. (2016). How the machine ‘thinks’: Understanding opacity in machine learning algorithms. Big Data & Society, 3(1), 1-12.
Janssen, M., & van den Hoven, J. (2015). Big and Open Linked Data (BOLD) in Government: A Challenge to Transparency and Privacy? Government Information Quarterly 32(4), 363–68.
Kemper, J. & Kolkman, D. (2018). Transparent to whom? No algorithmic accountability without a critical audience. Information, Communication & Society, 22(14), 2081-2096.
Kleinberg, J., Lakkaraju, H., Leskovec, J., Ludwig, J., & Mullainathan, S. (2017). Human decisions and machine predictions. The Quarterly Journal of Economics, 133(1), 237-293.
Kroll, J. A., Barocas, S., Felten, E. W., Reidenberg, J. R., Robinson, D. G., & Yu, H. (2016). Accountable algorithms. University of Pennsylvania Law Review, 165, 633.
Margetts, H., & Dorobantu, C. (2019). Rethink government with AI. Nature 568, 163-165.
Mittelstadt, B. D., Allo, P., Taddeo, M., Wachter, S., & Floridi, L. (2016). The ethics of algorithms: Mapping the debate. Big Data & Society, 3(2): 1-21.
Peeters, R., & Schuilenburg, M. (2018). Machine justice: Governing security through the bureaucracy of algorithms. Information Polity, 23(3), 267-280.
Pencheva, I., Esteve, M., & Mikhaylov, S. J. (2018). Big Data and AI–A transformational shift for government: So, what next for research?. Public Policy and Administration doi: 10.1177/0952076718780537
Schmidt, V.A. (2013). Democracy and Legitimacy in the European Union Revisited: Input, Output and ‘Throughput’. Political Studies, 61(1), 2-22.
Statistics Netherlands (CBS). Verkennend onderzoek naar het gebruik van algoritmen binnen overheidsorganisaties [exploratory investigation of the use of algorithms in government organizations]. https://www.cbs.nl/nl-nl/maatwerk/2018/48/gebruik-van-algoritmen-door-o… access Sep 10, 2019.
Whittaker, Meredith, Kate Crawford, Roel Dobbe, Genevieve Fried, Elizabeth Kaziunas, Varoon Mathur, Sarah Myers West, Rashida Richardson, Jason Schultz , Oscar Schwartz. 2018. AI Now report 2018, New York University